General Overview: The Applications Workshop and tutorials will be on July 19th. The main conference will run from the morning of July 20 through late afternoon/early evening on Sunday, July 22.
Invited Speakers
Speaker Overview:- Moises Goldszmidt, Making Life Better One Large Distributed System at a Time: Challenges for UAI Research
- James E. Smith, The Optimizer's Curse
- Ronald A. Rensink, The Challenge of Scene Perception
- Marco F. Ramoni, Statistical Mechanics of Biological Networks
- James V. Zidek, Computational Strategies for Modeling and Regulating Air Pollution Fields
Invited Talk I, Friday, July 20, 11:10 - 12:10
Moises Goldszmidt
Microsoft
Research Silicon Valley Lab
Making Life Better One Large Distributed System at a Time: Challenges for UAI Research
Abstract: Services in the internet are changing the way we travel, access information, invest, bank, shop, and conduct business and research. These services are supported by an ecosystem of information technology (IT), including storage, network, and middleware/applications that is growing in complexity. These IT systems present numerous management challenges both in terms of day to day operations, and in terms of strategic and long term planning. Instrumentation and measurement technology is, by and large, keeping pace with this development and growth. However, the algorithms, tools, and technology required to transform the data into relevant information for decision making are not. The claim in this talk is that the line of research conducted in Uncertainty in Artificial Intelligence is very well suited to address the challenges and close this gap. I will support this claim and discuss open problems using recent examples in diagnosis, model discovery, and policy optimization on three real life distributed systems.
Speaker Bio: Dr. Moises Goldszmidt is a principal researcher with Microsoft Research. His interests are in probabilistic representation and reasoning, decision-making, machine learning, pattern recognition, statistical inference, algorithms, artificial intelligence and the management of large systems. Since 1999, Moises has been focusing his research in the application of statistical pattern recognition and probabilistic reasoning to the diagnosis, forecasting, and control of performance problems and faults in complex networked systems. He has over 45 publications in his fields of interest, and several patents. He regularly participates as a program committee member in scientific meetings, was the co-chair of the Uncertainty in AI Conference (2000 and 2001), and was the co-chair of the ACM workshop on "applications of machine learning for tackling system's problems" (ACM-sysML 2006). Prior to Microsoft he held similar positions with Hewlett-Packard Labs, SRI International, and Rockwell Science Center, and was a principal scientist with Peakstone Corp. (start-up). Dr Goldszmidt has a PhD degree in Computer Science from UCLA (1992).
Invited Talk II: Saturday, July 21, 11:10 - 12:10
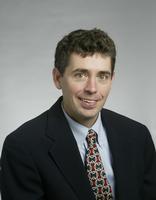
James E. Smith
The Fuqua School of Business, Duke University
The Optimizer's Curse
Abstract: In decision analysis, we build models to estimate the expected value or expected utility of various alternatives and then rank the alternatives by these value estimates. Computer systems tasked with making intelligent decisions follow a similar process and other intelligent systems provide estimates for use in such a decision-making process. With uncertainty and limited resources, a model is never perfect. Consequently, the value estimates are subject to error. In this talk, we show that if we take the value estimates at face value and select according to these estimates, we should expect the value of the chosen alternative to be less than its estimate, even if the value estimates are unbiased. Thus, when comparing actual outcomes to value estimates, we should expect to be disappointed on average, not because of any inherent bias in the estimates themselves, but because of the optimization-based selection process. We call this phenomenon the "optimizer's curse" and argue that it is not well understood or appreciated in the decision analysis, management science, and artificial intelligence communities. This curse may be a factor in creating skepticism in decision makers who review the results of an analysis or recommendations of an intelligent system. In this talk, we discuss the optimizer's curse and show that the resulting expected disappointment may be substantial. We then propose the use of Bayesian methods to adjust value estimates. These Bayesian methods can be viewed as disciplined skepticism and provide a method for avoiding this postdecision disappointment.
Based upon joint work with Robert L. Winkler at Duke University.
Speaker Bio: James E. Smith is a Professor in the Decision Sciences area at the Fuqua School of Business at Duke University. He teaches courses in probability and statistics and decision modeling. Professor Smith's research interests lie primarily in the areas of decision analysis and focus on developing methods for formulating and solving dynamic decision problems and valuing risky investments. His research has been supported by grants from the National Science Foundation, Chevron, and the Eli Lilly Foundation. He is currently a William and Sue Gross Distinguished Research Scholar.
Professor Smith received B.S. and M.S. degrees in Electrical Engineering from Stanford University (in 1984 and 1986) and worked as a management consultant prior to earning his Ph.D. in Engineering-Economic Systems at Stanford in 1990. He has been at Fuqua since the fall of 1990, and received the Outstanding Faculty Award from the daytime MBA students in 1993 and 2000. He served as Associate Dean for the Duke MBA Program from 2000-03. He won Fuqua's Bank of America award for outstanding research, teaching and service in 2004.
Outside of Duke, Smith has been a member of the Advisory Panel for the National Science Foundation's Decision Risk and Management Science program and on a pair of National Academy committees charged with estimating the benefits of the Department of Energy's investments in fossil energy and energy efficiency research and development. Smith has served in a variety of editorial roles including six years as the Departmental Editor for Decision Analysis at Management Science. He currently serves on the editorial board for Decision Analysis.
Banquet Speaker, Saturday, July 21, 20:00
Ronald A. Rensink
Department of Psychology, University of
British Columbia
The Challenge of Scene Perception
Abstract: Although it appears to us as observers that we always see everything in front of us, recent work in visual perception has shown that this is not true. For example, it has been found that observers have great difficulty noticing changes that occur during a brief interruption or eye movement, even if the changes are large and the observer expects them. This phenomenon of "change blindness" has motivated much investigation over the past decade into issues such as how much of a scene is remembered, what kinds of memory systems are involved, and what role is played by visual attention. Several of the more recent insights into the mechanisms involved will be discussed, including the proposal that scene perception is based on a dynamic "just-in-time" process, and that the successful coordination of this relies critically on a careful interplay between internal knowledge and external information. In addition, some proposals will be presented as to how techniques to handle uncertainty in AI might be adapted to provide the theoretical basis for a computational understanding of scene perception, one that is relevant to both humans and machines.
Speaker Bio: Ronald Rensink is an Associate Professor in the departments of Computer Science and Psychology at the University of British Columbia (UBC), Vancouver, Canada. His interests include human vision (particularly visual attention), computer vision, and human-computer interaction. He has presented work at major conferences on basic vision science, computer graphics, and consciousness. He obtained his PhD in Computer Science (specializing in computer vision) from UBC in 1992. He returned to UBC in 2000, and is currently part of the UBC Cognitive Systems Program, an interdisciplinary program that combines Computer Science, Linguistics, Philosophy, and Psychology.
Invited Talk III, Sunday, July 22, 11:10 - 12:10
Marco F. Ramoni
Biomedical Cybernetics Laboratory, Harvard University, and
Massachusetts Institute of Technology
Statistical Mechanics of Biological Networks
Abstract: Network models are today extensively used to encode and process biological information. Thanks to over two decades of research in artificial intelligence, statistics and decision theory, methods abound to automatically extract network models from large databases. As we become faster at generating data and smarter at building networks from them, we are rewarded with larger and larger networks. These networks, which may contain tens of thousand of nodes connected by hundreds of thousand of links, are too large to visually inspect and too complex to manually explore. Therefore, understanding the information encoded in these networks requires the ability to automatically analyze their topological structure. This talk will describe a statistical mechanics approach to the topological analysis of large-scale networks. It will show that such topological analyses can identify empirically testable hypothesis and, in the field of biological networks, predict functional properties of living systems. The talk will illustrate how these methods can be used for a wide range of tasks, from the identification of the genes involved in the control mechanisms of tumor growth to the prediction of the effectiveness of anti-cancer compounds.
Speaker Bio: Marco F Ramoni is Assistant Professor of Pediatrics and Medicine (Bioinformatics) at Harvard Medical School and Assistant Professor of Health Sciences and Technology at the Harvard-MIT Division of Health Sciences and Technology. He is also the director of the Biomedical Cybernetics Laboratory at the Harvard-Partners Center for Genetics and Genomics, where he serves as Associate Director of Bioinformatics, and the Director of the Training Fellowship in Biomedical Informatics at Children's Hospital Boston. He is co-founder of Bayesware LLC, a software company developing Artificial Intelligence programs based on Bayesian methods, and Phorecaster Inc, a company developing predictive models for drug development. He received a PhD in Biomedical Engineering and a BA in Philosophy (Epistemology) from the University of Pavia (Italy) and his postdoctoral training from McGill University, Montreal (Canada).
Invited Talk IV, Sunday, July 22, 14:00 - 15:00

James V. Zidek
Department of Statistics, University of British Columbia
Computational Strategies for Modeling and Regulating Air Pollution Fields
Abstract: The earth's atmosphere is a complex stochastic system which includes amongst other things pollution fields, a part of each deriving from anthropogenic sources and activities. Because of their negative health impacts, these fields are now subject to regulation. However setting the air quality standards needed to regulate them is itself a complex business and that leads to a need for good models for these fields. This talk, drawing on the speaker's recent experience and research connected with ozone, will describe physical, computational and statistical approaches to modeling pollution fields and how these might be combined. Finally he will describe some of the ways in which the results of these models play into the process of developing standards. Although focusing on random pollution fields, the modeling issues have become quite pervasive in current research in statistical science.
Speaker Bio: James V Zidek, Professor Emeritus and Founding Head of Statistics at the University of British Columbia, obtained his PhD at Stanford University. His interests include statistical decision analysis and environmental statistics, having made both theoretical and applied contributions to the latter. Service includes being President of the Statistical Society of Canada (SSC). Honors include the SSC's Gold Medal and Fellowship in the Royal Society of Canada. Among other current appointments he serves on the the EPA's CASAC Ozone Review Panel.